报告题目:Policy Learning in Adaptive Experiments
报告时间:2023-04-20 10:00-11:00
报告人:Prof.Ruochan Zhan, Hong Kong University of Science and Technology
报告地点:理学院东北楼三楼会议室
Abstract: Learningg optimal policies from historical data enables personalization in a variety of domains across healthcare, digital recommendations, and online education. Recently, there has been increasing attention on adaptive experiments (for example, contextual bandits), which allow for progressively updating data-collection rules to identify good treatment assignment policies. However, most existing contextual bandit algorithms are geared towards maximizing the operational performance during the experiment, while the optimality of the learned policy is yet to be guaranteed, especially when outcome models are misspecified. Conversely, non-adaptive experiments, known as randomized controlled trials (RCT), guarantee to identify the best policy in large samples but can be prohibitively costly or even unethical in some cases. We propose to address this policy learning problem from two perspectives:
Offline policy learning using adaptively collected data. We seek to make the fullest use of such data, which is increasingly prevalent due to the popularity of adaptive designs, so as to learn a policy—without doing new experiments—that yields the best outcome for each individual. We show that our algorithm is robust to model misspecification and achieves the minimax optimality, even when the data is collected by an original experiment with diminishing exploration.
Online contextual bandit algorithm tailored to policy learning. We seek to design a practical contextual bandit algorithm to collect “relevant” data for policy learning, such that our algorithm guarantees to learn the optimal policy at a faster rate than RCT in many instances. We also show that our algorithm can be flexibly adapted to optimize the performance during the experiment (a.k.a. cumulative regret minimization) with minimax optimality guarantees.
The talk is based on joint works with Susan Athey, Emma Brunskill, Sanath Krishnamurthy, Zhimei Ren, and Zhengyuan Zhou.
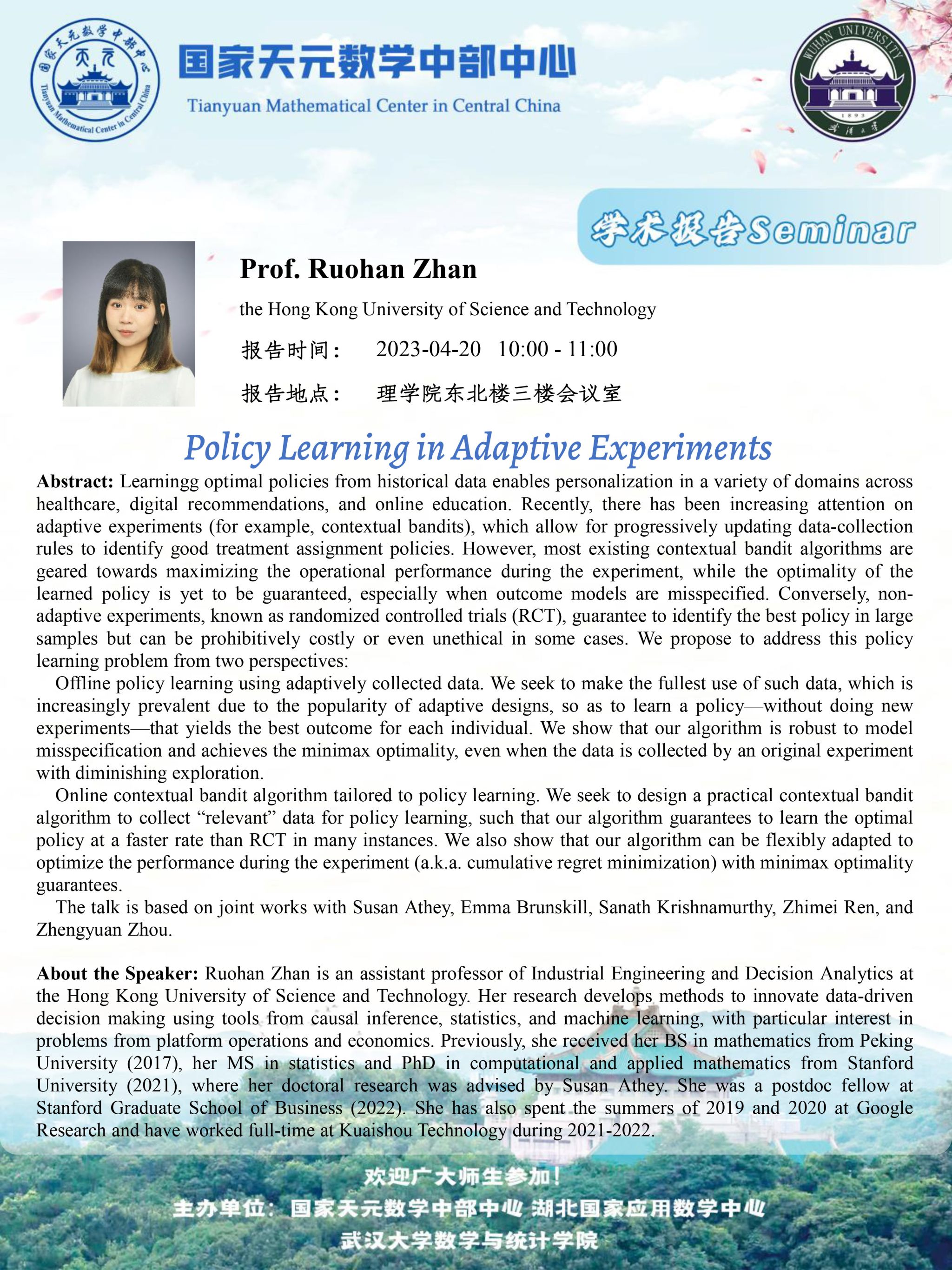