报告题目:Response Best-Subset Selector for Large-Scale Response Variables
报告时间:2021-11-02 16:30-17:30
报告人:刘 旭 上海财经大学
腾讯会议ID:422 415 983
访问此链接进入会议,或添加至会议列表:https://meeting.tencent.com/dm/MFy3Mquqh213
Abstract: This article investigates the statistical problems of response variable selection with exponentially large-scale response variables for multivariate linear regression with two settings of fixed and diverging numbers of predictor variables. A response best-subset selection model is proposed by introducing a 0-1 selection indictor to each response variable, and the response best-subset selector is developed, which is an efficient procedure for performing response variable selection and regression coefficient estimation simultaneously by introducing a separation parameter and a novel penalty function. The proposed response best-subset selectors for two settings both have consistency under mild conditions. For the fixed number of predictor variables, consistency and asymptotic normality are presented for the corresponding regression coefficient estimators. The Bonferroni test procedure with F-type test statistics turns out to be a special case of our response best-subset selector. In finite-sample simulation studies our response best-subset selector has stronger competitive advantages in keeping balance between higher accurate rates of important and unimportant response variables or larger Matthews correlation coefficient over its main competitors. A real data analysis demonstrates the effectiveness of the response best-subset selector for identifying dosage-sensitive genes.
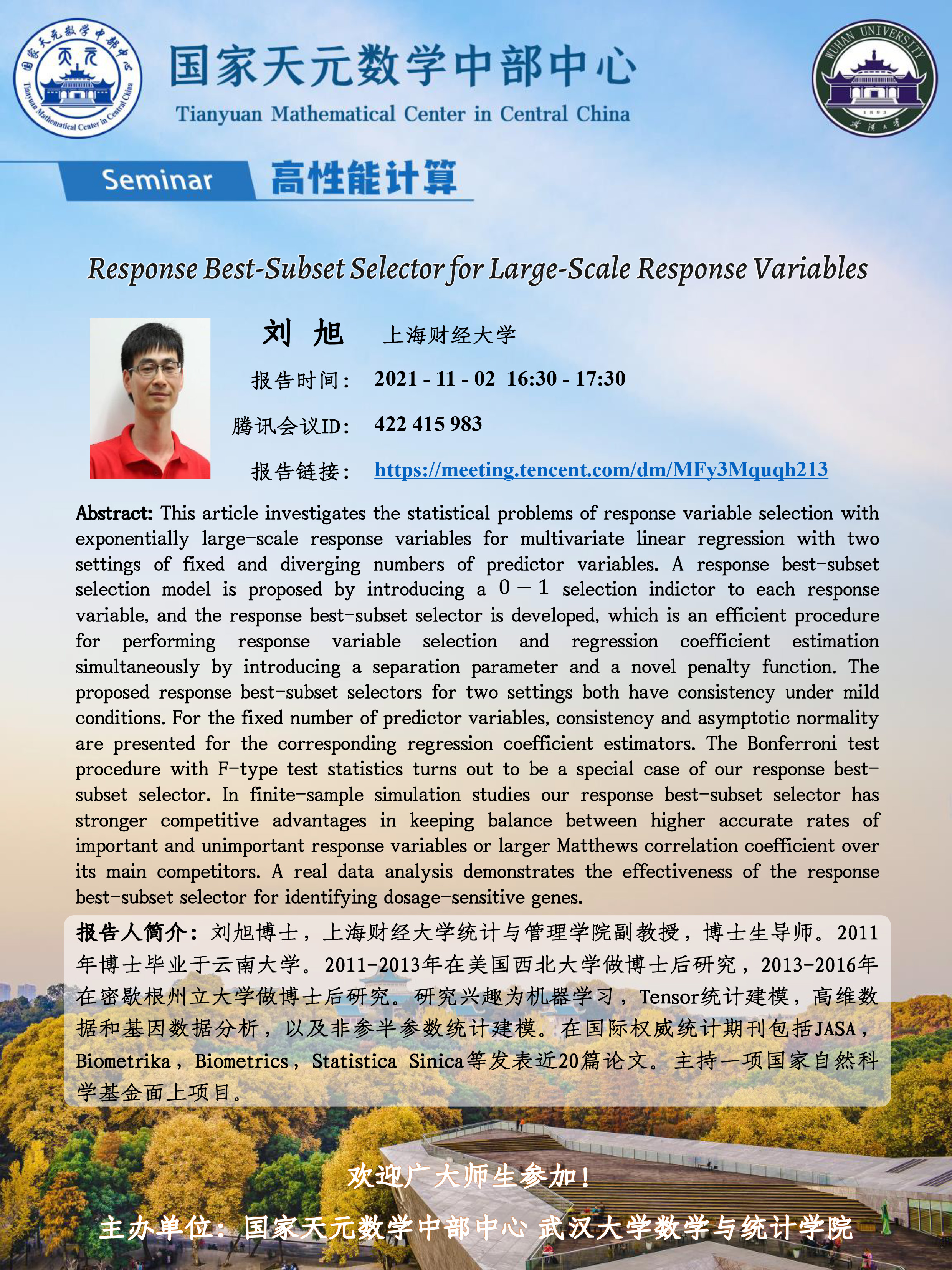